Enhancing Public Safety and Service Satisfaction – A Local Government’s NPS & Key Driver Analysis
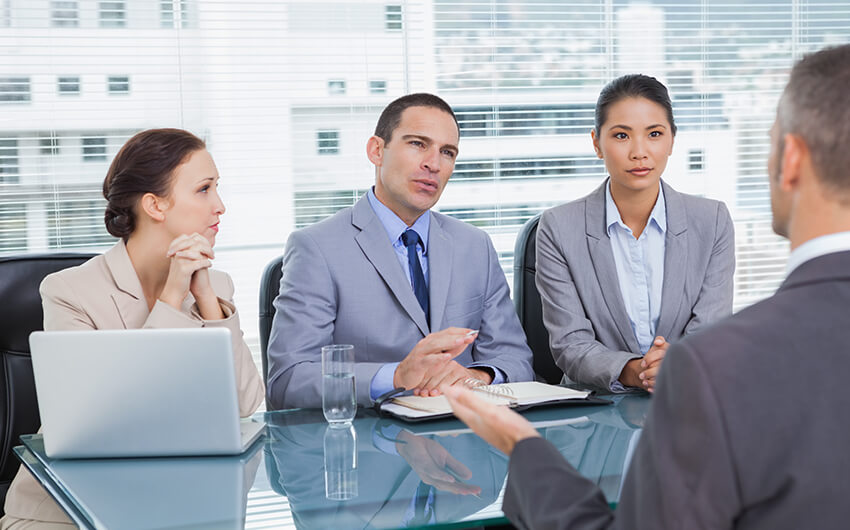
Client Overview
A local government agency responsible for public safety, community services, and infrastructure faced declining public satisfaction based on Net Promoter Score (NPS) surveys. The agency had conducted periodic surveys to assess resident sentiment on safety, emergency response, public works, and other municipal services, but lacked the data-driven insights to:
1.
Identify the key drivers influencing public perception of safety and municipal services.
The local government sought a data science-driven solution to analyze NPS trends, uncover key drivers, and implement changes that would increase community satisfaction while optimizing city resources.
Phase 1: Data Collection & Understanding the Public Sentiment
Survey Data & NPS Calculation
The local government had conducted surveys quarterly to gauge public sentiment on safety and municipal services. Questions covered:
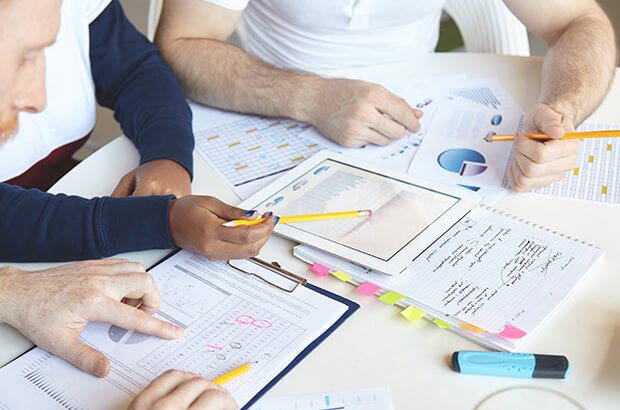
Perception of safety (police presence, emergency response time).
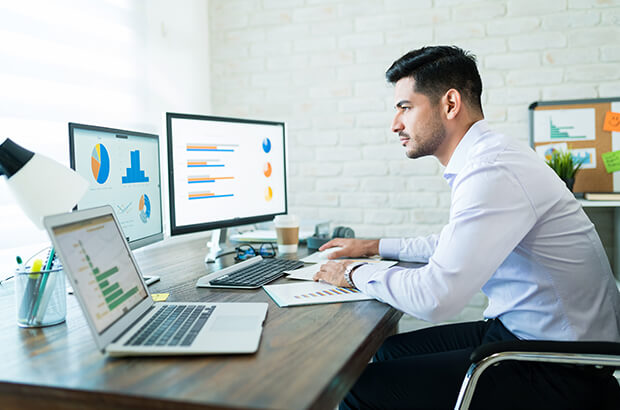
Phase 2: Applying Data Science for Key Driver Analysis
The government agency partnered with BI Consulting Services to apply advanced data science techniques to extract meaningful insights from survey data and drive improvements.
Machine Learning-Based Key Driver Analysis
To determine the primary factors impacting NPS, we used:
Phase 3: Implementing Data-Driven Solutions
1.
Improving Emergency Response Time
Predictive analytics for emergency dispatch optimization:
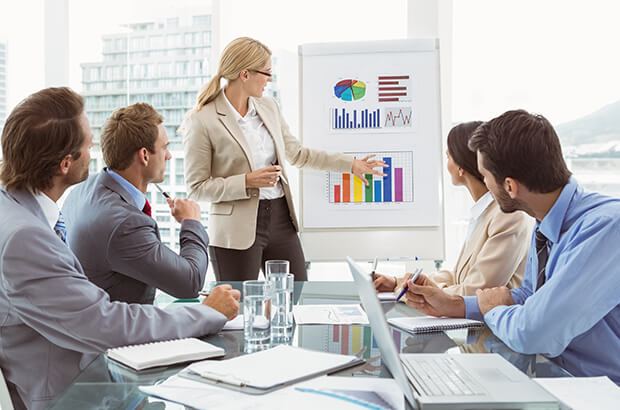
2.
Enhancing Public Safety Infrastructure
Smart street lighting project:
3.
AI-Powered Citizen Reporting System
AI chatbot & mobile app for complaints:
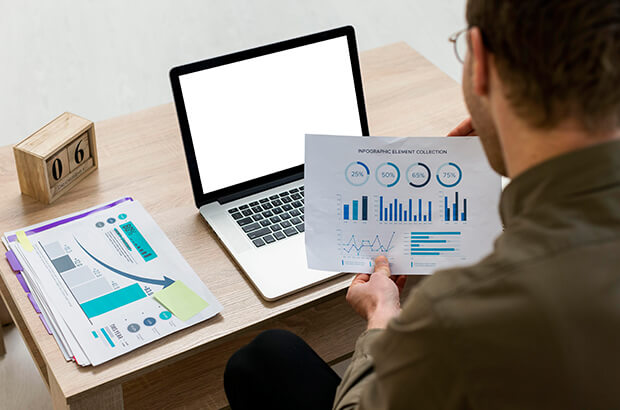
Results & Impact
NPS increased from -10 to +15 in six months.
Emergency response time improved by 18%, boosting resident confidence.
Public works complaint resolution time reduced by 40%, leading to a cleaner, safer environment.
Crime perception improved by 22%, leading to greater community engagement with local government.